Predictive Analytics: Forecasting the Future with Data
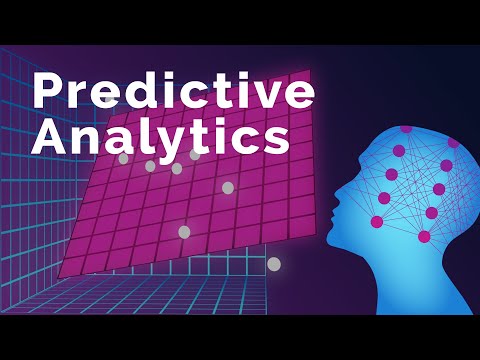
Hello readers, how are you? Welcome to an exciting journey into the world of predictive analytics, where we unlock the power of data to forecast the future. In this article, we will delve into the fascinating realm of data-driven insights and explore how they shape our understanding of what lies ahead. So, whether you're a seasoned data enthusiast or just beginning to dip your toes into the vast ocean of analytics, greetings and welcome! Please continue reading to uncover the secrets behind predictive analytics and how it can revolutionize the way we perceive and navigate the future.
Introduction to Predictive Analytics
Predictive analytics is a powerful tool that helps businesses make informed decisions by analyzing historical data to predict future outcomes. By using various statistical techniques and machine learning algorithms, predictive analytics can identify patterns, trends, and relationships within data sets, enabling organizations to anticipate customer behavior, optimize marketing campaigns, manage risk, and improve operational efficiency.
This advanced analytical approach plays a vital role in fields such as finance, healthcare, marketing, and manufacturing. With the increasing availability of big data and advancements in technology, predictive analytics is becoming more accessible and valuable for businesses of all sizes.
By harnessing the power of predictive analytics, organizations can gain a competitive edge, make proactive decisions, and drive growth in today's data-driven world.
Understanding the Role of Data in Predictive Analytics
Data plays a vital role in predictive analytics. By harnessing the power of data, organizations can gain valuable insights and make informed decisions. Predictive analytics involves the use of statistical algorithms and machine learning techniques to analyze historical data and make predictions about future events or outcomes.
It enables businesses to anticipate customer behavior, optimize operations, and mitigate risks. Data is the fuel that drives predictive analytics, providing the necessary information to build accurate models and make accurate predictions.
Without high-quality data, predictive analytics would be ineffective. Therefore, understanding the role of data in predictive analytics is crucial for organizations seeking to leverage its potential and stay ahead in today's data-driven world.
The Importance of Forecasting in Business Decision-Making
Forecasting plays a crucial role in the decision-making process of businesses. By predicting future trends and outcomes, it enables companies to make informed choices and develop effective strategies.
Without accurate forecasting, businesses may face significant risks and uncertainties. The ability to anticipate market demand, identify potential opportunities, and mitigate potential risks is essential for success in today's competitive landscape.
Forecasting helps businesses allocate resources efficiently, optimize production, and manage inventory levels effectively. It also aids in financial planning and budgeting, ensuring that businesses can meet their financial goals.
Ultimately, forecasting empowers businesses to make proactive decisions, stay ahead of the curve, and thrive in an ever-changing marketplace. Its importance cannot be overstated as it provides the foundation for strategic decision-making and long-term business growth.
Key Concepts and Techniques in Predictive Analytics
Predictive analytics is a powerful tool that enables businesses to make informed decisions based on historical data. By analyzing patterns, trends, and correlations, predictive analytics can forecast future outcomes and help organizations identify potential risks and opportunities.
Key concepts in predictive analytics include data collection, data preparation, model building, and model evaluation. Data collection involves gathering relevant data from various sources such as databases, social media, and customer surveys.
Once the data is collected, it needs to be cleaned and transformed into a format suitable for analysis.Data preparation involves tasks such as data cleansing, feature selection, and data transformation.
This step is crucial in ensuring the accuracy and quality of the data used for analysis. Model building is the process of creating a predictive model using statistical techniques and algorithms. There are various modeling techniques, including regression, classification, and clustering.
Once the model is built, it needs to be evaluated to assess its accuracy and effectiveness. Model evaluation techniques include cross-validation, hypothesis testing, and performance metrics such as precision, recall, and F1 score.
In addition to these key concepts, there are various techniques used in predictive analytics, such as time series analysis, decision trees, neural networks, and ensemble methods. These techniques allow businesses to uncover hidden patterns and relationships in their data, enabling them to make accurate predictions and optimize their decision-making process.
Overall, predictive analytics plays a crucial role in helping businesses gain a competitive edge by leveraging data to make data-driven decisions. By understanding the key concepts and techniques in predictive analytics, organizations can harness the power of data to drive business growth and success.
Exploring Different Types of Predictive Models
Exploring different types of predictive models is a fascinating endeavor in the field of data science. These models play a crucial role in making accurate predictions based on historical data and patterns.
From simple linear regression to complex ensemble methods like random forests and gradient boosting, there is a wide range of predictive models to choose from. Each model has its own strengths and weaknesses, making it essential to understand their nuances before applying them to real-world scenarios.
Whether it's classification, regression, or time series forecasting, having a solid understanding of the different types of predictive models empowers data scientists to make informed decisions and extract valuable insights from their data.
Data Collection and Preparation for Predictive Analytics
Data collection and preparation are crucial steps in the process of predictive analytics. With the advancement of technology and the increasing availability of data, organizations have access to vast amounts of information that can be used to make informed decisions and gain valuable insights.
However, the effectiveness of predictive analytics relies heavily on the quality and accuracy of the collected data. This involves identifying relevant data sources, cleaning and transforming the data, and ensuring its integrity.
Additionally, data preparation includes feature engineering, where variables are selected or created to enhance the predictive models. By carefully collecting and preparing data, organizations can unlock the power of predictive analytics and make more accurate predictions to drive their business forward.
Feature Selection and Engineering in Predictive Analytics
Feature selection and engineering are crucial steps in the field of predictive analytics. These processes involve the careful selection and transformation of variables to enhance the accuracy and performance of predictive models.
Traditional approaches often rely on human intuition and domain expertise to identify relevant features. However, as the volume and complexity of data continue to increase, automated techniques have gained popularity.
These methods leverage algorithms and statistical techniques to identify the most informative features from a large pool of variables. Feature engineering, on the other hand, involves creating new features or transforming existing ones to capture more meaningful information.
This can include techniques like one-hot encoding, scaling, or creating interaction terms. The goal is to create a feature set that maximizes the predictive power of the model. Both feature selection and engineering play a vital role in improving the performance and interpretability of predictive models, making them essential components in the field of predictive analytics.
Model Training and Evaluation in Predictive Analytics
In the field of predictive analytics, model training and evaluation play a crucial role in ensuring accurate and reliable predictions. Model training involves feeding data into a machine learning algorithm, allowing it to learn patterns and relationships within the data.
This process enables the algorithm to make predictions based on new, unseen data. Once the model is trained, it needs to be evaluated to assess its performance. Evaluation metrics such as accuracy, precision, recall, and F1 score are commonly used to measure the model's effectiveness.
By iteratively training and evaluating models, predictive analytics practitioners can refine their models and improve their predictive capabilities.
Overcoming Challenges in Predictive Analytics Implementation
Overcoming Challenges in Predictive Analytics Implementation can be a daunting task for organizations. The successful integration of predictive analytics requires careful planning, robust infrastructure, and skilled professionals.
One of the main challenges is the availability and quality of data. Often, organizations struggle to gather relevant and accurate data to feed into their predictive models. Additionally, data privacy and security concerns pose significant barriers, as organizations must ensure that sensitive information is protected throughout the analytics process.
Another challenge lies in selecting the right algorithms and models that align with the organization's goals and objectives. This requires expertise in data science and a deep understanding of the business context.
Finally, organizational resistance to change can hinder the implementation process. Stakeholders may be skeptical or resistant to adopting predictive analytics, making it crucial to communicate the benefits and address any concerns.
By addressing these challenges head-on, organizations can unlock the full potential of predictive analytics and gain valuable insights to drive informed decision-making.
Ethical Considerations in Predictive Analytics
Predictive analytics is a powerful tool that uses data and algorithms to forecast future outcomes and trends. However, it also raises important ethical considerations that must not be overlooked. One key concern is privacy.
Predictive analytics relies on vast amounts of personal data, and there is a risk of this information being misused or mishandled. Another issue is bias. The algorithms used in predictive analytics are only as good as the data they are trained on, and if that data contains biases, the predictions will also be biased.
This can lead to unfair discrimination in areas such as hiring or lending decisions. Transparency is another ethical consideration. Users should have a clear understanding of how predictive analytics works and how their data is being used.
Lastly, there is the question of accountability. Who is responsible if a prediction goes wrong or if someone is unfairly affected by the results? These ethical considerations highlight the need for careful regulation and responsible use of predictive analytics to ensure that its potential benefits are realized without sacrificing individual rights and fairness.
Post a Comment for "Predictive Analytics: Forecasting the Future with Data"